4 Key Risks of Implementing AI: Real-Life Examples & Solutions
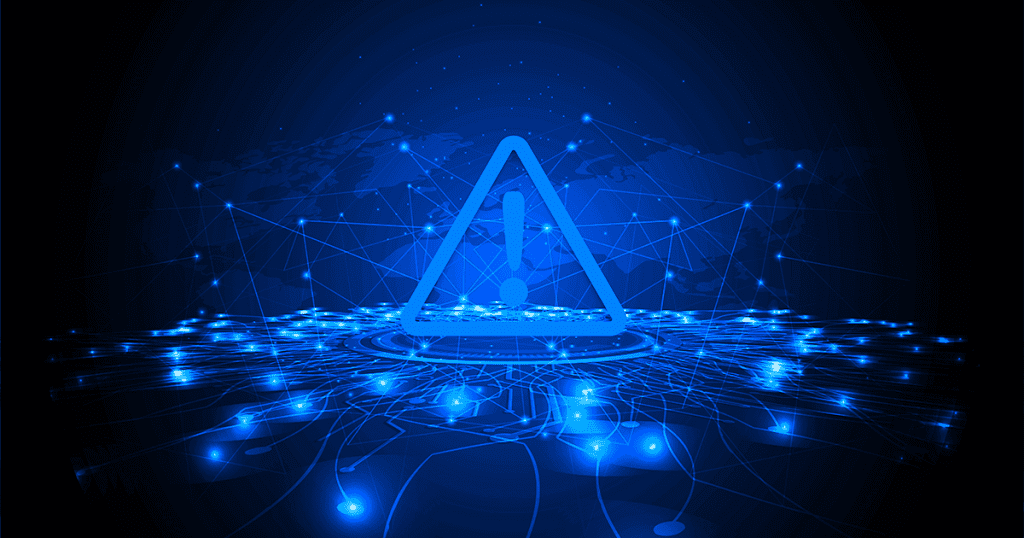
As artificial intelligence (AI) adoption gathers pace, so does the complexity and range of its risks. Businesses are increasingly aware of these challenges, yet the roadmaps to solutions often remain shrouded in obscurity.
If the question ‘How to navigate these risks?’ resonates with you, then this article will serve as a lighthouse in the fog. We delve into the heart of AI’s most pressing issues, bolstered by real-life instances, and lay out clear, actionable strategies to safely traverse this intricate terrain.
Read on to unlock valuable insights that could empower your business to leverage the potency of AI, all the while deftly sidestepping potential pitfalls.
1. Bias in AI-Based Decisions
The unintentional inclusion of bias in AI systems is a significant risk with far-reaching implications. This risk arises because these systems learn and form their decision-making processes based on the data they are trained on.
If the datasets used for training include any form of bias, these prejudices will be absorbed and consequently reflected in the system’s decisions.
Example: Algorithmic Bias in the UK A-level Grading
To illustrate, consider a real-world example that occurred during the COVID-19 pandemic in the UK. With the traditional A-level exams canceled due to health concerns, the UK government used an algorithm to determine student grades.
The algorithm factored in various elements, such as a school’s historical performance, student subject rankings, teacher evaluations, and past exam results. However, the results were far from ideal.
Almost 40% of students received grades lower than expected, sparking widespread backlash. The primary issue was the algorithm’s over-reliance on historical data from schools to grade individual students.
If a school hadn’t produced a student who achieved the highest grade in the past three years, no student could achieve that grade in the current year, regardless of their performance or potential.
This case demonstrates how algorithmic bias can produce unjust and potentially damaging outcomes.
Possible Solution: Human-in-the-loop Approach
So, how can we avoid this pitfall? The answer lies in human oversight. It’s essential to keep humans involved in AI decision-making processes, especially when these decisions can significantly impact people’s lives.
While AI systems can automate many tasks, they should not completely replace human judgment and intuition.
Sectors Where Sole Reliance on AI Decisions Should Be Avoided
The so-called human-in-the-loop approach is especially crucial in sectors where AI-based decisions directly impact individual lives and society.
These sectors include:
- Education: As demonstrated by the UK, AI systems should not be solely responsible for grading assignments or predicting students’ academic performance. A human teacher’s expertise and personal understanding of their students should play a decisive role in these situations.
- Healthcare: AI has made significant strides in disease diagnosis, treatment planning, and patient care. However, the potential for misdiagnosis or inadequate treatment planning due to biases or errors in AI systems emphasizes the necessity of human professionals in the final decision-making process.
- Recruitment and HR: AI is increasingly being used for resume screening and predicting potential job performance. However, reliance on AI can lead to biased hiring practices, potentially overlooking candidates with unconventional backgrounds or skill sets. A human-in-the-loop approach ensures comprehensive and fair candidate evaluation.
- Finance and Lending: AI algorithms can evaluate creditworthiness, but they may inadvertently discriminate based on geographical location or personal spending habits, which can correlate with ethnicity or socioeconomic status. In such scenarios, human judgment is necessary to ensure balanced lending decisions.
- Criminal Justice: AI is being used to predict crime hotspots and potential reoffending. However, bias in historical crime data can lead to unjust profiling and sentencing. Human oversight can provide more nuanced perspectives and help prevent such injustices.
- Autonomous Vehicles: Though AI drives the operation of self-driving cars, it’s crucial to have a human in the decision-making process, especially when the vehicle has to make ethical decisions in scenarios of unavoidable accidents.
2. Violating Personal Privacy
In the rapidly evolving digital world, data has become a pivotal resource that drives innovation and strategic decision-making.
The International Data Corporation predicts that the global datasphere will swell from 33 zettabytes in 2018 to a staggering 175 zettabytes by 2025. However, this burgeoning wealth of data also escalates the risks associated with personal privacy violations.
As this datasphere expands exponentially, the potential for exposing sensitive customer or employee data increases in lockstep. And when data leaks or breaches occur, the fallout can be devastating, leading to severe reputational damage and potential legal ramifications, particularly with tighter data processing regulations being implemented across the globe.
Example: Samsung’s Data Breach with ChatGPT
A vivid illustration of this risk can be seen in a recent Samsung incident. The global tech leader had to enforce a ban on ChatGPT when it was discovered that employees had unintentionally revealed sensitive information to the chatbot.
According to a Bloomberg report, proprietary source code had been shared with ChatGPT to check for errors, and the AI system was used to summarize meeting notes. This event underscored the risks of sharing personal and professional information with AI systems.
It served as a potent reminder for all organizations venturing into the AI domain about the paramount importance of solid data protection strategies.
Possible Solutions: Data anonymization & More
One critical solution to such privacy concerns lies in data anonymization. This technique involves removing or modifying personally identifiable information to produce anonymized data that cannot be linked to any specific individual.
Companies like Google have made data anonymization a cornerstone of their privacy commitment. By analyzing anonymized data, they can create safe and beneficial products and features, such as search query auto-completion, all while preserving user identities. Furthermore, anonymized data can be shared externally, allowing other entities to benefit from this data without putting user privacy at risk.
However, data anonymization should be just one part of a holistic data privacy approach that includes data encryption, strict access controls, and regular data usage audits. Together, these strategies can help organizations navigate the complex landscape of AI technologies without jeopardizing individual privacy and trust.
[ Read also: 6 Essential Tips to Enhance Your Chatbot Security in 2023 ]
3. Opacity and Misunderstanding in AI Decision Making
Artificial intelligence is riddled with complexities, made all the more acute by the enigmatic nature of many AI algorithms.
As prediction-making tools, the inner workings of these algorithms can be so intricate that comprehending how the myriad variables interact to produce a prediction can challenge even their creators. This opacity, often called the ‘black box’ dilemma, has been a focus of investigation for legislative bodies seeking to implement appropriate checks and balances.
Such complexity in AI systems and the associated lack of transparency can lead to distrust, resistance, and confusion among those using these systems. This problem becomes particularly pronounced when employees are unsure why an AI tool makes specific recommendations or decisions and could lead to reluctance to implement the AI’s suggestions.
Possible solution: Explainable AI
Fortunately, a promising solution exists in the form of Explainable AI. This approach encompasses a suite of tools and techniques designed to make the predictions of AI models understandable and interpretable. With Explainable AI, users (your employees, for example) can gain insight into the underlying rationale for a model’s specific decisions, identify potential errors, and contribute to the model’s performance enhancement.
Example: An EdTech Organization Leveraging Explainable AI for Trustworthy Recommendations
The DLabs.AI team successfully employed this approach during a project for a global EdTech platform. We developed an explainable recommendation engine, enabling the student support team to understand why the software recommended specific courses.
Explainable AI allowed us and our client to dissect decision paths in decision trees, detect subtle overfitting issues, and refine data enrichment. This transparency in understanding the decisions made by ‘black box’ models fostered increased trust and confidence among all parties involved.
4. Unclear Legal Responsibility
Artificial Intelligence’s rapid advancement has resulted in unforeseen legal issues, especially when determining accountability for an AI system’s decisions. The complexity of the algorithms often blurs the line of responsibility between the company using the AI, the developers of the AI, and the AI system itself.
Example: Uber Self-Driving Car Incident
A real-world case highlighting the challenge is a fatal accident involving an Uber self-driving car in Arizona in 2018. The car hit and killed Elaine Herzberg, a 49-year-old pedestrian wheeling a bicycle across the road. This incident marked the first death on record involving a self-driving car, leading to Uber discontinuing its testing of the technology in Arizona.
Investigations by the police and the US National Transportation Safety Board (NTSB) primarily attributed the crash to human error. The vehicle’s safety driver, Rafael Vasquez, was found to have been streaming a television show at the time of the accident. Although the vehicle was self-driving, Ms. Vasquez could take over in an emergency. Therefore, she was charged with negligent homicide while Uber was absolved from criminal liability.
Solution: Legal Frameworks & Ethical Guidelines for AI
To address the uncertainties surrounding legal liability for AI decision-making, it’s necessary to establish comprehensive legal frameworks and ethical guidelines that account for the unique complexities of AI systems.
These should define clear responsibilities for the different parties involved, from developers and users to companies implementing AI. Such frameworks and guidelines should also address the varying degrees of autonomy and decision-making capabilities of different AI systems.
For example, when an AI system makes a decision leading to a criminal act, it could be considered a “perpetrator via another,” where the software programmer or the user could be held criminally liable, similar to a dog owner instructing their dog to attack someone.
Alternatively, in scenarios like the Uber incident, where the AI system’s ordinary actions lead to a criminal act, it’s essential to determine whether the programmer knew this outcome was a probable consequence of its use.
The legal status of AI systems could change as they evolve and become more autonomous, adding another layer of complexity to this issue. Hence, these legal frameworks and ethical guidelines will need to be dynamic and regularly updated to reflect the rapid evolution of AI.
Conclusion: Balancing Risk and Reward
As you can see, AI brings numerous benefits but also involves significant risks that require careful consideration.
By partnering with an experienced advisor specializing in AI, you can navigate these risks more effectively. We can provide tailored strategies and guidance on minimizing potential pitfalls, ensuring your AI initiatives adhere to transparency, accountability, and ethics principles. If you’re ready to explore AI implementation or need assistance managing AI risks, schedule a free consultation with our AI experts. Together, we can harness the power of AI while safeguarding your organization’s interests.
latest post
ReplyDelete